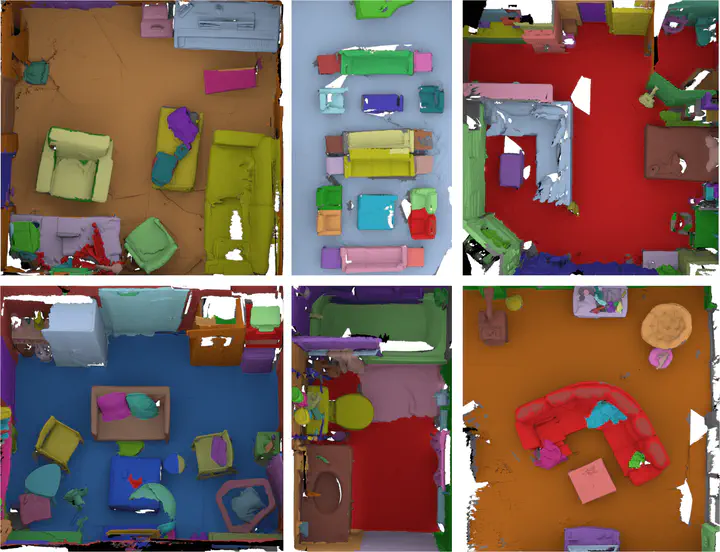
In this work, we take VoteNet, a state-of-the-art deep neural network for object detection, as a basis and extend it for computing epistemic uncertainty in itspredictions with Monte Carlo dropout. We show that this quantity can be used as a weighting factor for predictions, leading to an increase in the overall performance ofthe model, and also as a metric for selecting the most beneficial scenes to annotate for training from unknown data for active learning scenarios.
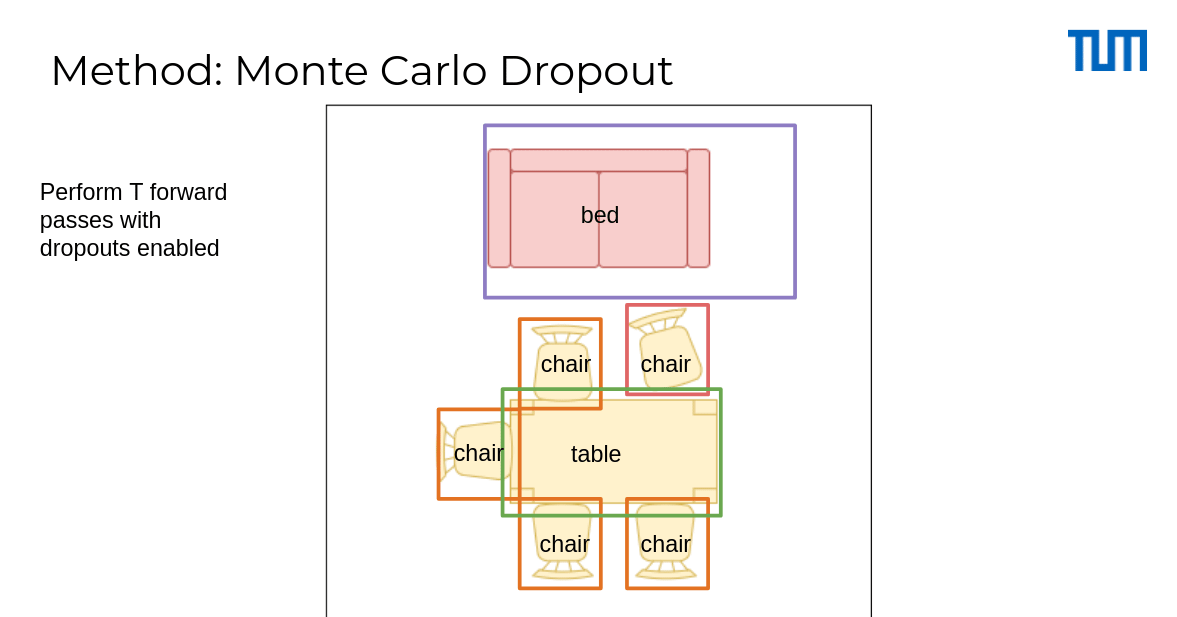