Probabilistic Object Detection and Reconstruction from a single RGB-D frame
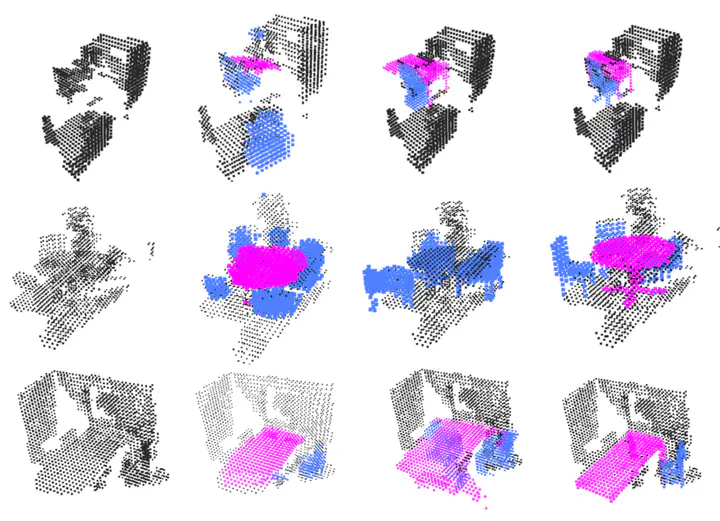
Semantic scene understanding is crucial aspect of modern day robotic applications. With the recent advances in deep learning and the increased availability of large scale richly annotated datasets, popularity of 3D scene understanding tasks has increased rapidly. In this work, we present a probabilistic hybrid solution with point-based and volumetric components to jointly localize, classify and complete the object instances given a single RGB-D frame. Our reconstructions are not restricted by the camera field of view, and aims to complete the object geometry even outside the camera frustum where the input signal is significantly weaker compared to the rest of the scene. Our probabilistic detection approach aims to combat ambiguous scenarios where objects in the scene have weak representation, and multiple completions are plausible for an object. We show that instead of outputting directly regressed bounding boxes, learning a distribution of bounding boxes can help us generate alternative suggestions for every detection proposal, which improves both detection and completion performance when utilized.
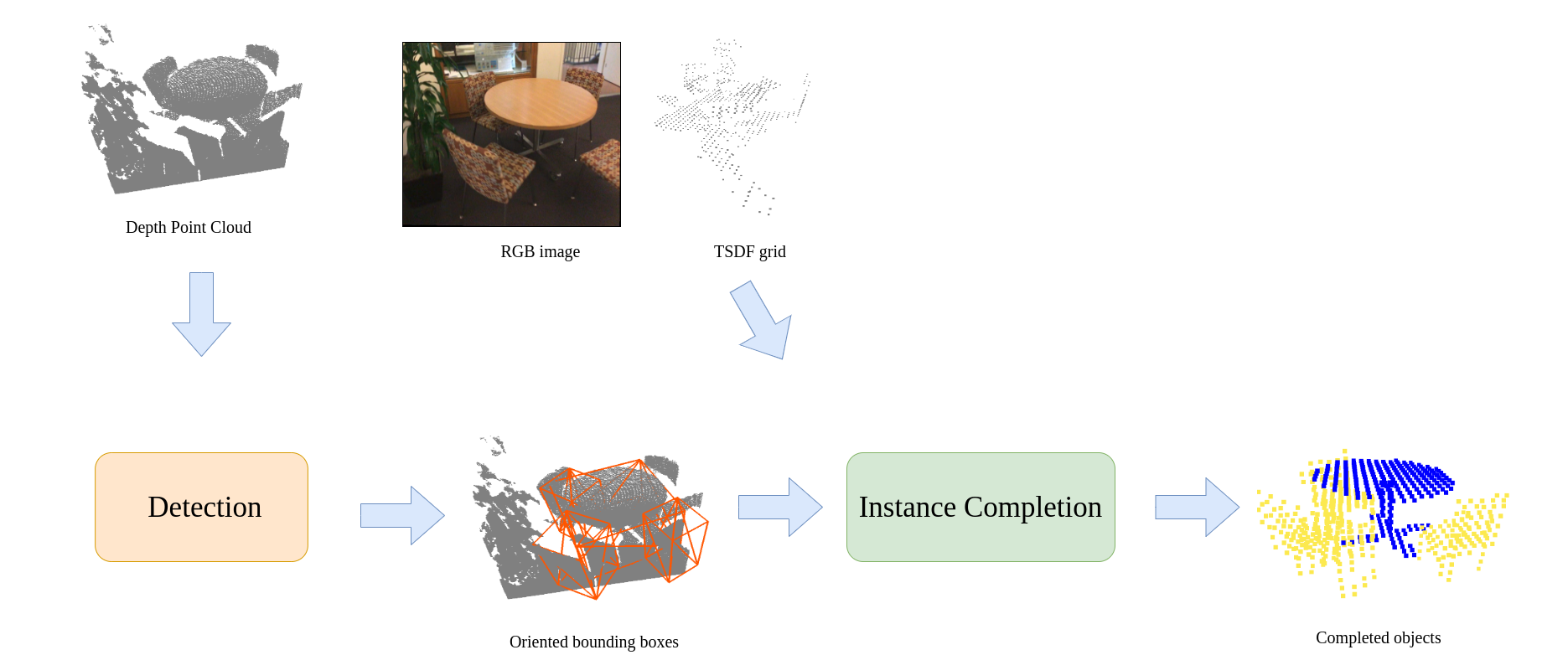
After performing detection and learning the bounding box distributions, we can sample multiple completion options for objects, one example is the following.
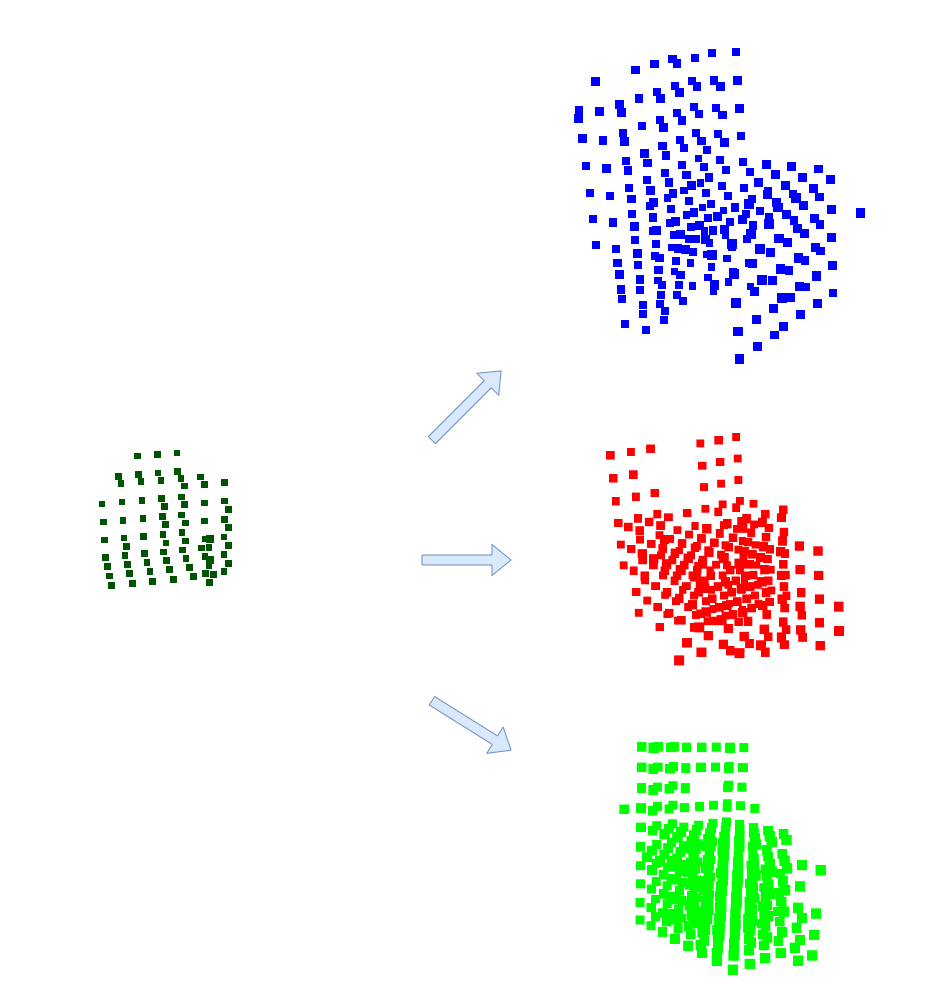